Note
You can download this example as a Jupyter notebook or start it in interactive mode.
Network Clustering#
In this example, we show how pypsa can deal with spatial clustering of networks.
[1]:
import pypsa
import re
import numpy as np
import matplotlib.pyplot as plt
import cartopy.crs as ccrs
import pandas as pd
ERROR 1: PROJ: proj_create_from_database: Open of /home/docs/checkouts/readthedocs.org/user_builds/pypsa/conda/latest/share/proj failed
[2]:
n = pypsa.examples.scigrid_de()
n.calculate_dependent_values()
WARNING:pypsa.io:Importing network from PyPSA version v0.17.1 while current version is v0.27.1. Read the release notes at https://pypsa.readthedocs.io/en/latest/release_notes.html to prepare your network for import.
INFO:pypsa.io:Imported network scigrid-de.nc has buses, generators, lines, loads, storage_units, transformers
The important information that pypsa needs for spatial clustering is in the busmap
. It contains the mapping of which buses should be grouped together, similar to the groupby groups as we know it from pandas.
You can either calculate a busmap
from the provided clustering algorithms or you can create/use your own busmap.
Cluster by custom busmap#
Let’s start with creating our own. In the following, we group all buses together which belong to the same operator. Buses which do not have a specific operator just stay on its own.
[3]:
groups = n.buses.operator.apply(lambda x: re.split(" |,|;", x)[0])
busmap = groups.where(groups != "", n.buses.index)
The clustering routine will raise an error if values in non-standard columns are not the same when combined to a common cluster. Therefore, we adjust the columns of the components and delete problematic non-standard values.
[4]:
n.lines = n.lines.reindex(columns=n.components["Line"]["attrs"].index[1:])
n.lines["type"] = np.nan
n.buses = n.buses.reindex(columns=n.components["Bus"]["attrs"].index[1:])
n.buses["frequency"] = 50
Now we cluster the network based on the busmap.
[5]:
C = n.cluster.get_clustering_from_busmap(busmap)
C
is a Clustering object which contains all important information. Among others, the new network is now stored in that Clustering object.
[6]:
nc = C.network
We have a look at the original and the clustered topology
[7]:
fig, (ax, ax1) = plt.subplots(
1, 2, subplot_kw={"projection": ccrs.EqualEarth()}, figsize=(12, 12)
)
plot_kwrgs = dict(bus_sizes=1e-3, line_widths=0.5)
n.plot(ax=ax, title="original", **plot_kwrgs)
nc.plot(ax=ax1, title="clustered by operator", **plot_kwrgs)
fig.tight_layout()
/home/docs/checkouts/readthedocs.org/user_builds/pypsa/conda/latest/lib/python3.11/site-packages/cartopy/mpl/feature_artist.py:144: UserWarning: facecolor will have no effect as it has been defined as "never".
warnings.warn('facecolor will have no effect as it has been '
/home/docs/checkouts/readthedocs.org/user_builds/pypsa/conda/latest/lib/python3.11/site-packages/cartopy/mpl/feature_artist.py:144: UserWarning: facecolor will have no effect as it has been defined as "never".
warnings.warn('facecolor will have no effect as it has been '
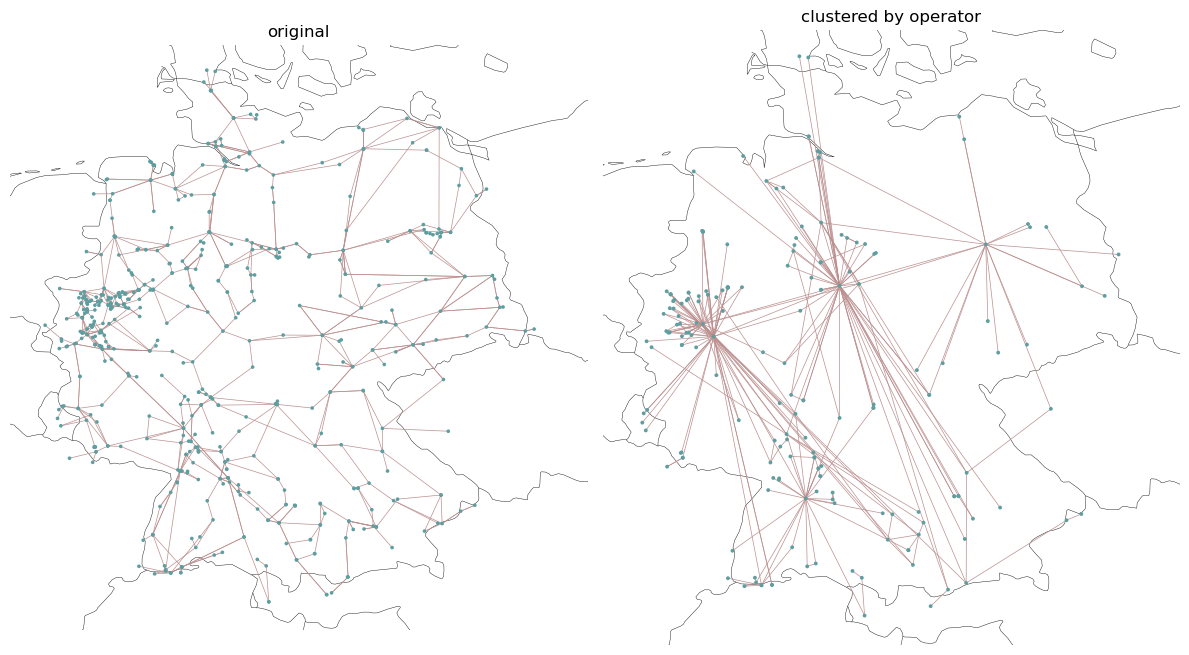
Looks a bit messy as over 120 buses do not have assigned operators.
Clustering by busmap created from K-means#
Let’s now make a clustering based on the kmeans algorithm. Therefore we calculate the busmap
from a non-weighted kmeans clustering.
[8]:
weighting = pd.Series(1, n.buses.index)
busmap2 = n.cluster.busmap_by_kmeans(bus_weightings=weighting, n_clusters=50)
We use this new kmeans-based busmap
to create a new clustered method.
[9]:
nc2 = n.cluster.cluster_by_busmap(busmap2)
Again, let’s plot the networks to compare:
[10]:
fig, (ax, ax1) = plt.subplots(
1, 2, subplot_kw={"projection": ccrs.EqualEarth()}, figsize=(12, 12)
)
plot_kwrgs = dict(bus_sizes=1e-3, line_widths=0.5)
n.plot(ax=ax, title="original", **plot_kwrgs)
nc2.plot(ax=ax1, title="clustered by kmeans", **plot_kwrgs)
fig.tight_layout()
/home/docs/checkouts/readthedocs.org/user_builds/pypsa/conda/latest/lib/python3.11/site-packages/cartopy/mpl/feature_artist.py:144: UserWarning: facecolor will have no effect as it has been defined as "never".
warnings.warn('facecolor will have no effect as it has been '
/home/docs/checkouts/readthedocs.org/user_builds/pypsa/conda/latest/lib/python3.11/site-packages/cartopy/mpl/feature_artist.py:144: UserWarning: facecolor will have no effect as it has been defined as "never".
warnings.warn('facecolor will have no effect as it has been '
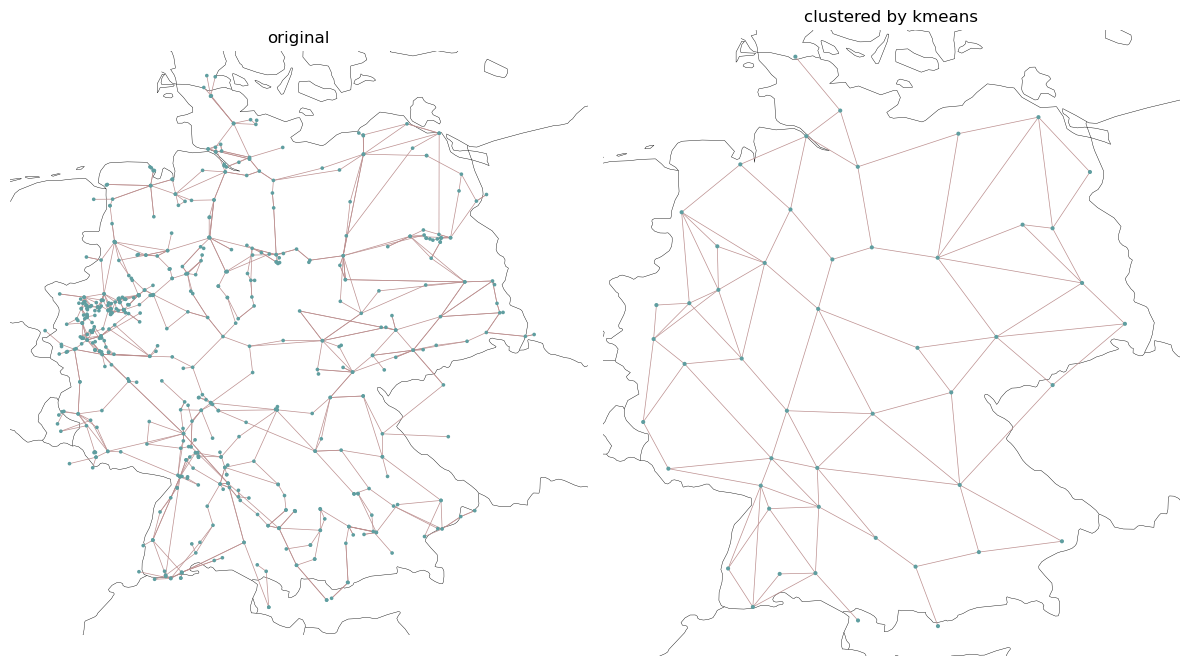
There are other clustering algorithms in the pipeline of pypsa as the hierarchical clustering which performs better than the kmeans. Also the get_clustering_from_busmap
function supports various arguments on how components in the network should be aggregated.